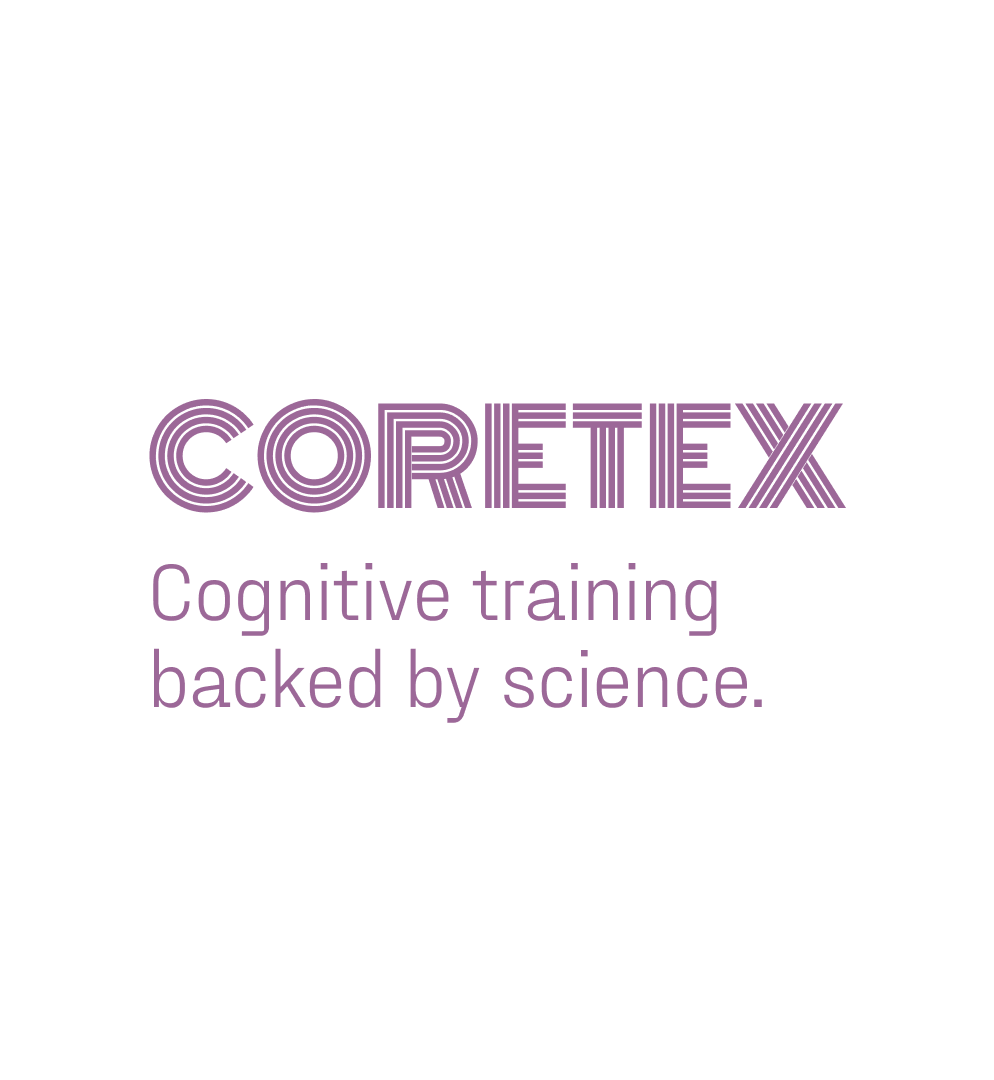
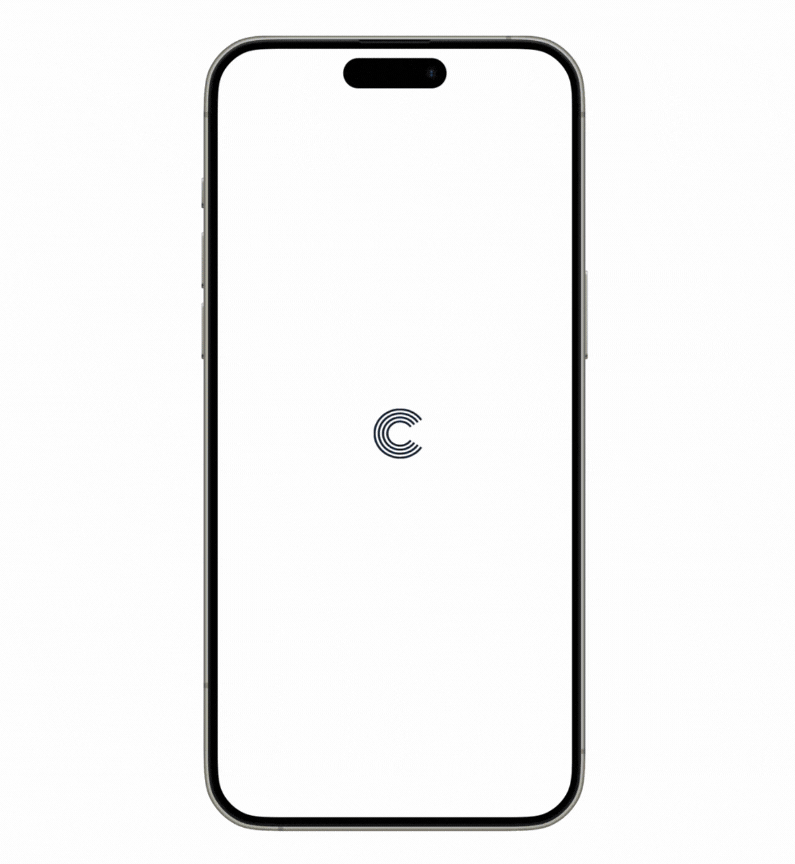
CORETEX
COGNITIVE TRAINING BACKED BY SCIENCE
CORETEX is an AI-native cognitive training platform designed to make preventive brain health proactive, engaging, and accessible. By transforming clinically-validated cognitive assessments into daily exercises, CORETEX creates a bridge between scientific validity and user engagement. The platform uses a proprietary reinforcement learning to personalize cognitive training across four key domains - Memory, Verbal, Movement, and Spatial awareness - while maintaining clear boundaries between wellness support and medical assessment.
Project Type
UX Research, UX Design, Digital Health
UX Research, UX Design, Digital Health
Team
2 Neuroscientists, 4 Engineers, 2 Designers
2 Neuroscientists, 4 Engineers, 2 Designers
Company
Kolachalama Laboratory, Boston University
Kolachalama Laboratory, Boston University
Year/Duration
May - Septemeber 2024, 16 weeks
May - Septemeber 2024, 16 weeks
Role
UX Researcher, UX Designer
UX Researcher, UX Designer
Tools
Figma
Figma
Skills
Research, Testing, Rapid Prototyping, Interaction Design
Research, Testing, Rapid Prototyping, Interaction Design
PROBLEM SPACE
Digital Health
Today's cognitive health landscape presents significant barriers for adults 45+, with prohibitive costs, long wait times, and limited access to preventive care. Traditional solutions either feel overly clinical or too game-like, while existing digital tools fail to maintain engagement or scientific validity, leaving users without effective options for maintaining cognitive wellness.
RESEARCH QUESTION
How Might We
How might we create an accessible and engaging cognitive training platform that effectively combines clinical validity with personalized user experience for adults 45+, addressing the growing need for preventive cognitive health tools?
SOLUTION
AI-Native Cognitive Training
To address these challenges and democratize cognitive health support, we developed CORETEX, an AI-native cognitive training platform that transforms clinical assessments into engaging daily exercises.
DISCOVER
Problem Space, User Research, Market Research, Landscape Audit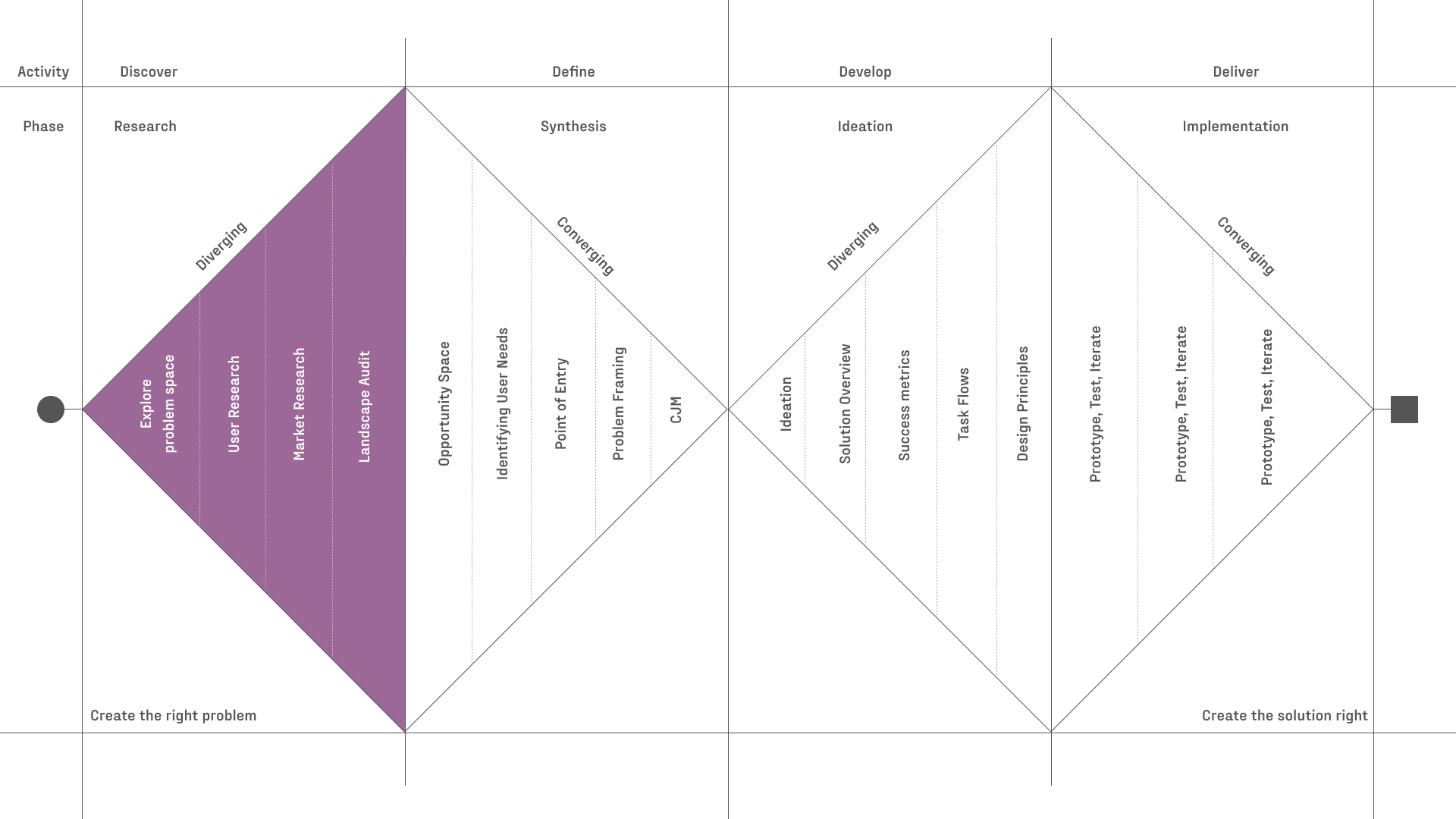
DISCOVER
Problem Space
Identifying the gap in the current state of the cognitive healthcare market.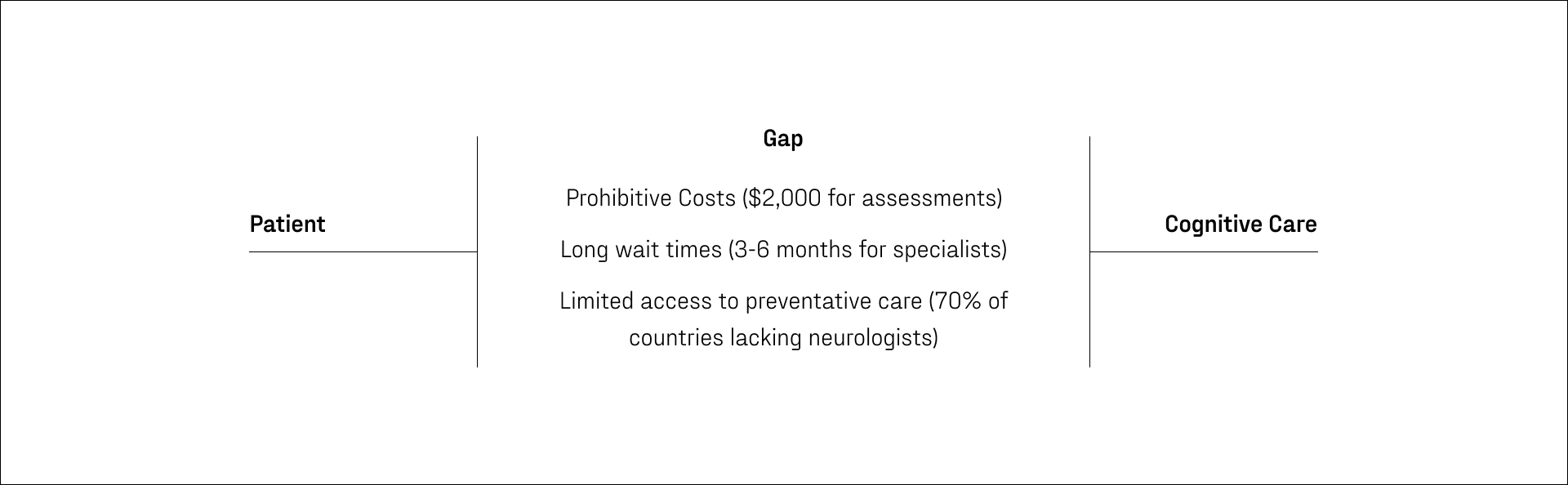
DISCOVER
User Research
Identifying the target audience as 45+ working professionals and their key motivators and concerns regarding cognitive healthcare.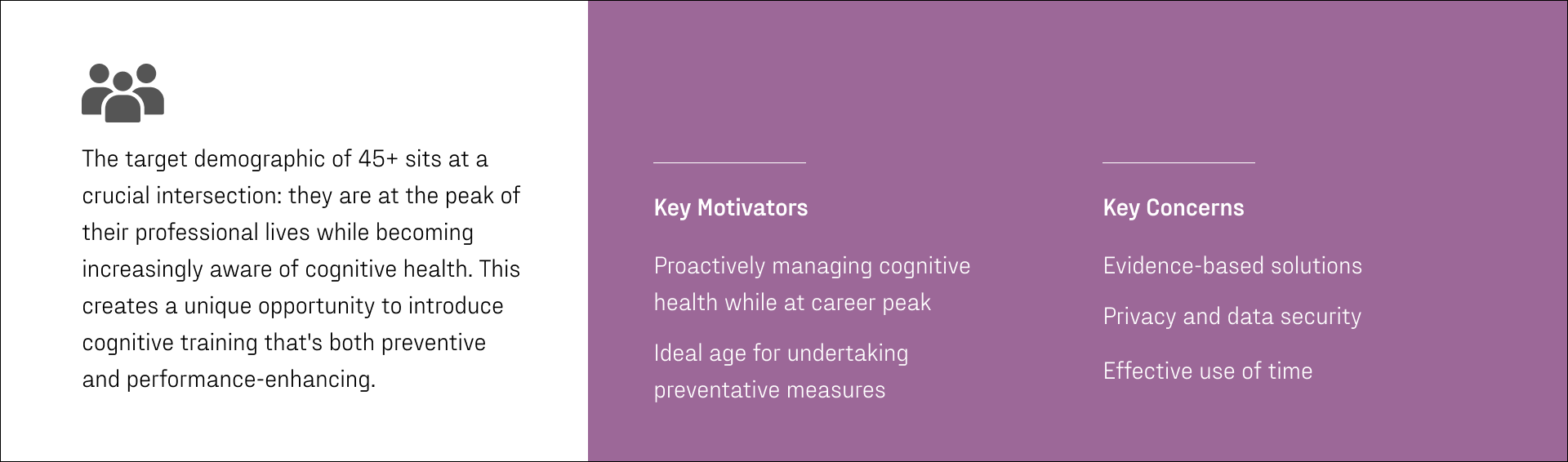
DISCOVER
Market Research
Undestanding the cognitive healthcare market through the perspective of changing demographics, longevity interventions, and digital interventions. 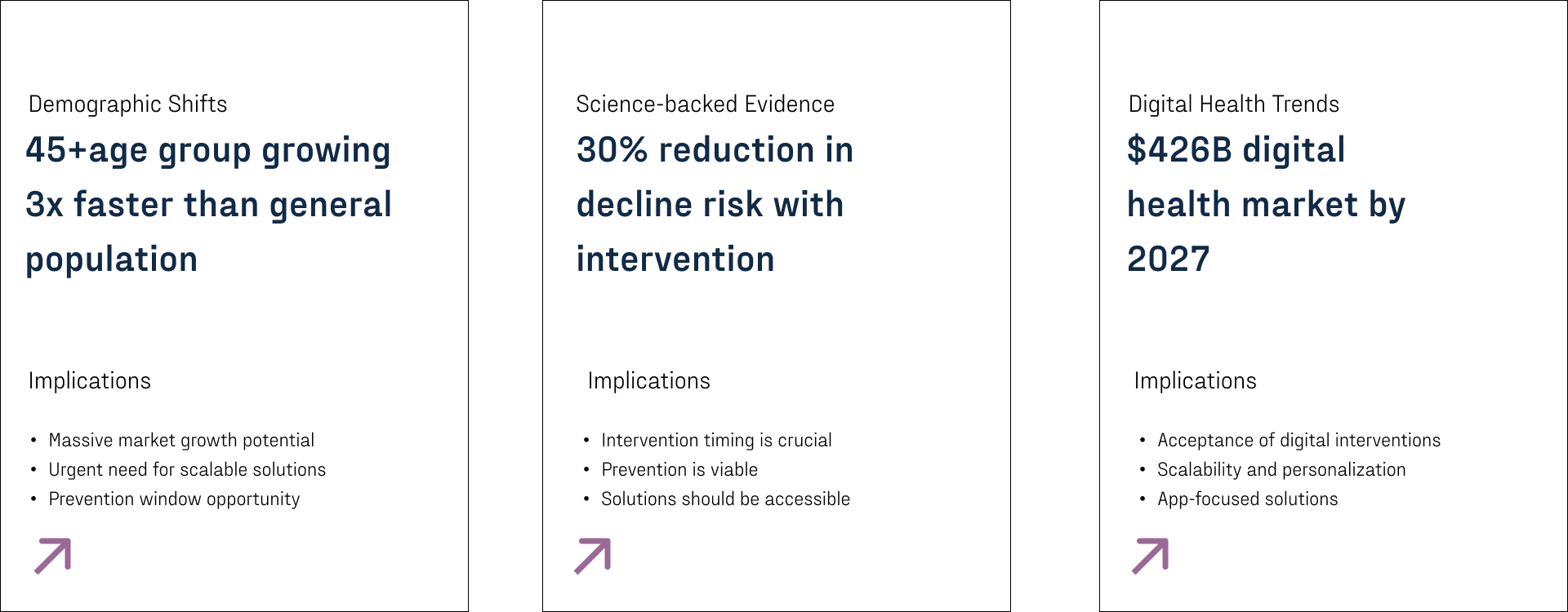
DISCOVER
Landscape Audit
Analysing popular word games, pattern-based puzzles, and established brain-training apps based on features and triggers that help them maintain their stickiness and market favor.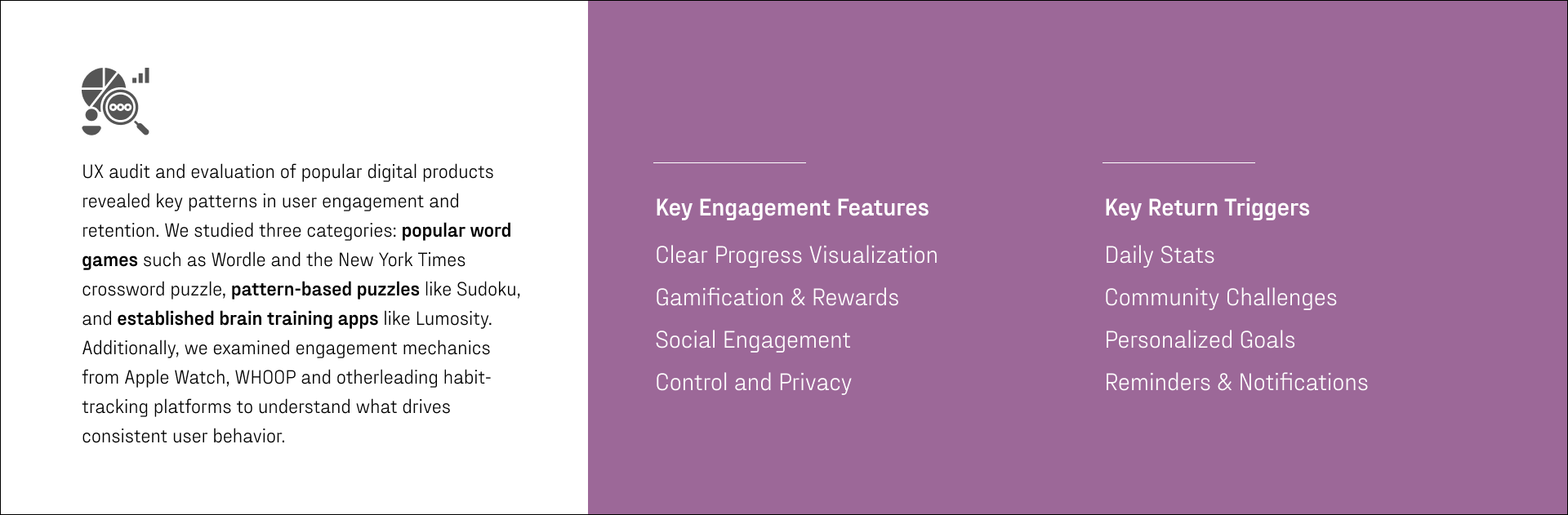
DEFINE
Opportunity Space, User Needs, Point of Entry, Problem Framing, Customer Journey Map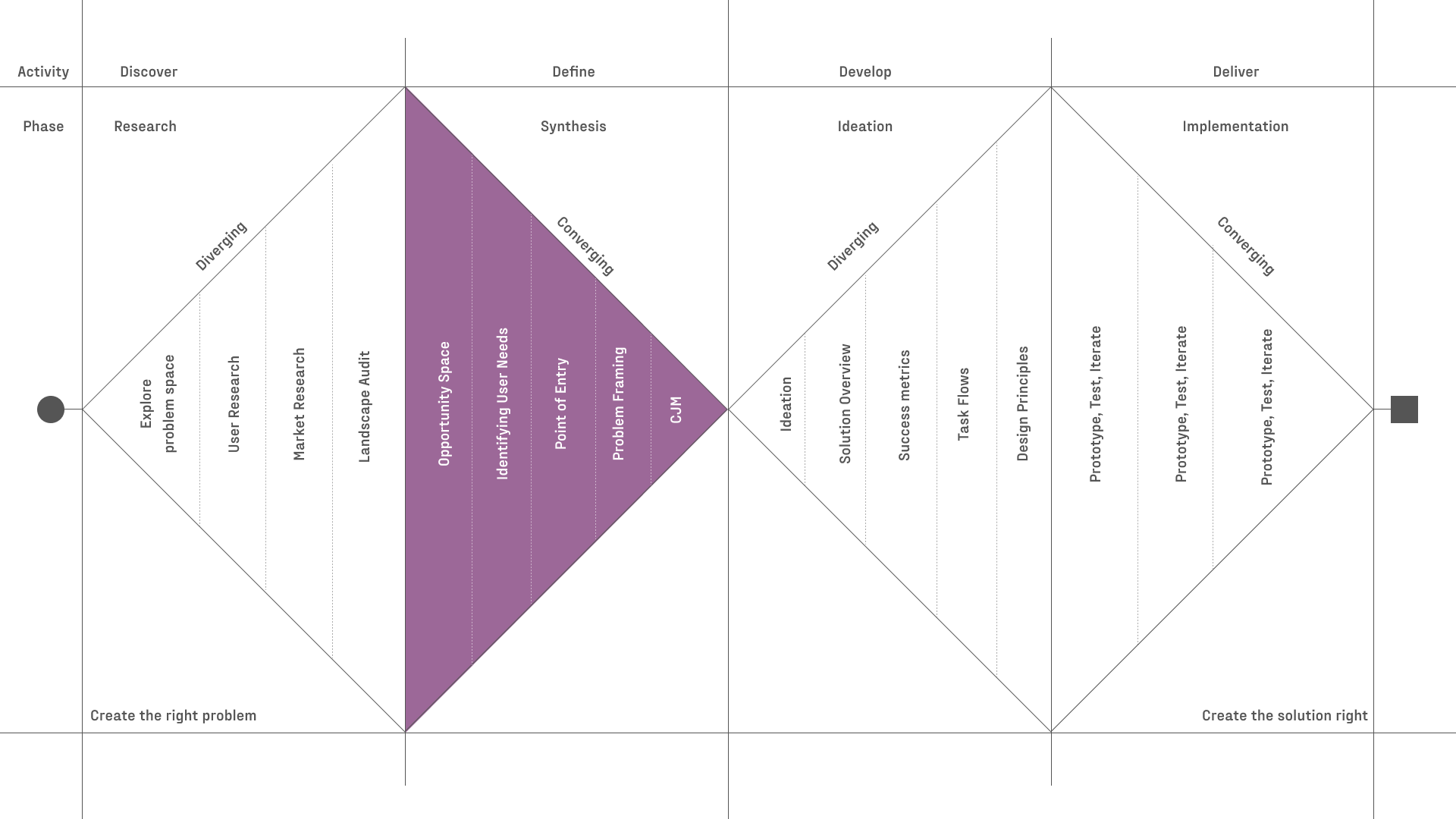
DEFINE
Opportunity Space
Establishing the angle with which to approach the problem of closing the gap between patients and accessible cognitive health care measures.
DEFINE
Jobs To Be Done
Identifying the functional, emotional, and social needs the target audience holds with regards to cognitive health monitoring.
DEVELOP
Problem Statement
Framing the how might we statement that guides the subsequent research and design process.︎
How might we create an accessible and engaging cognitive training platform that effectively combines clinical validity with personalized user experience for adults 45+, addressing the growing need for preventitive cognitive health tools?
︎
DEVELOP
Ideation, Solution Overview, Success Metrics, Task Flows, Design Principles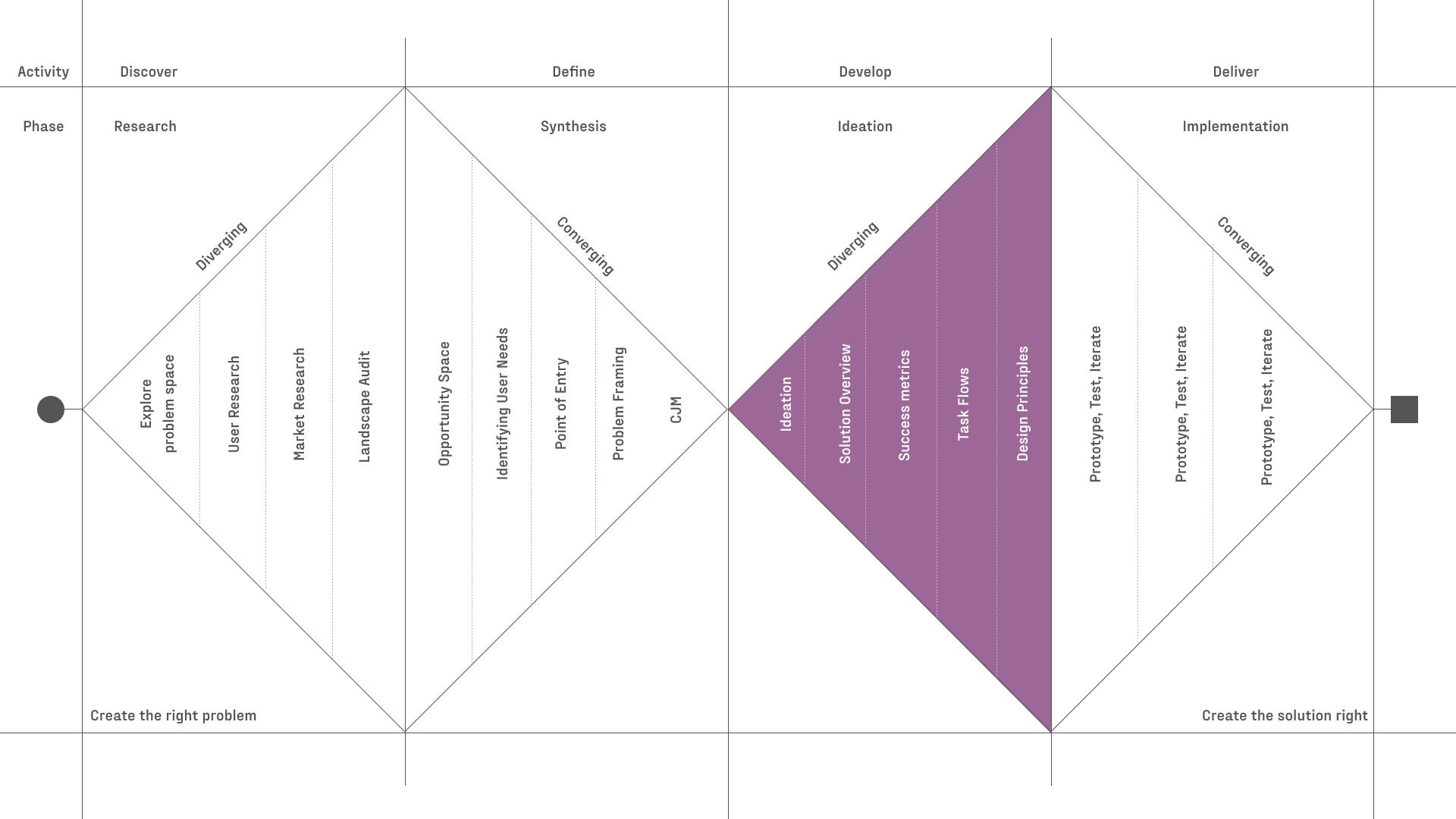
DEVELOP
Feature Prioritization
Brainstorming and validating the primary features required for the MVP based on discussed metrics such as NPS, usability surveys, task completion rate, and more.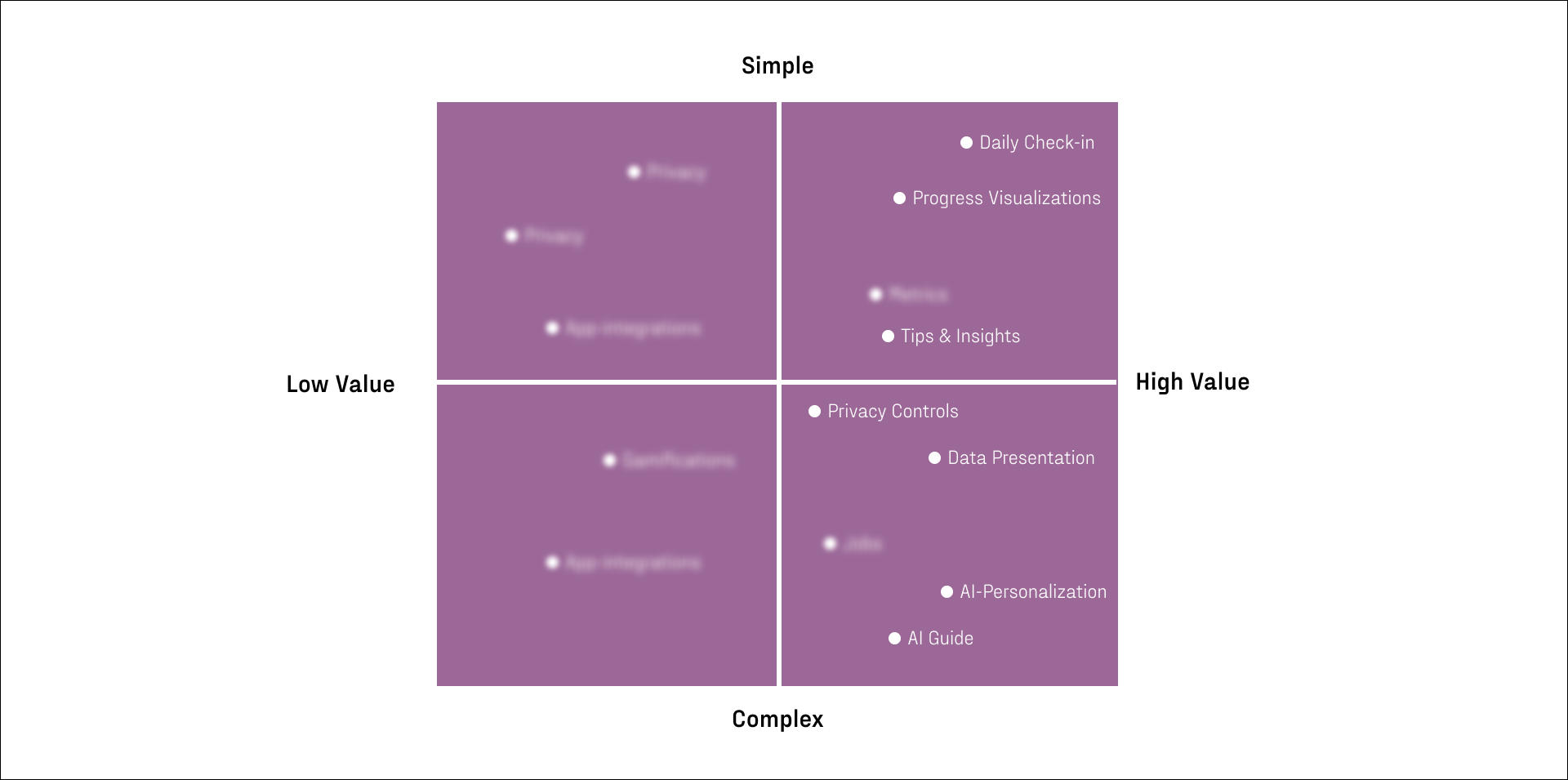
DEVELOP
Solution Statment
︎
CORETEX is an AI-enhanced cognitive training platform that transforms traditional clinical assessements into engaging daily activites.
︎
DEVELOP
Design Principles
To ensure users felt in control of their experience while helping them understand the involvement of the reinforcement learning (RL) model, these key principles - developed from insights from the research process - were implemented to design for transparency around the RL model.
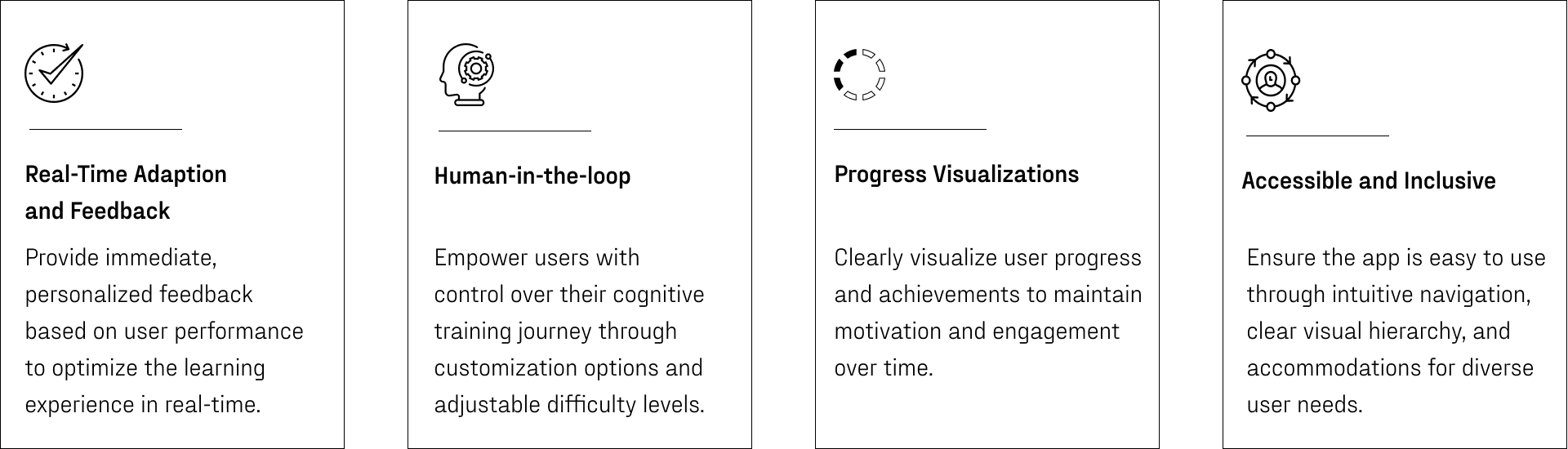
DELIVER
Prototype, Test, Iterate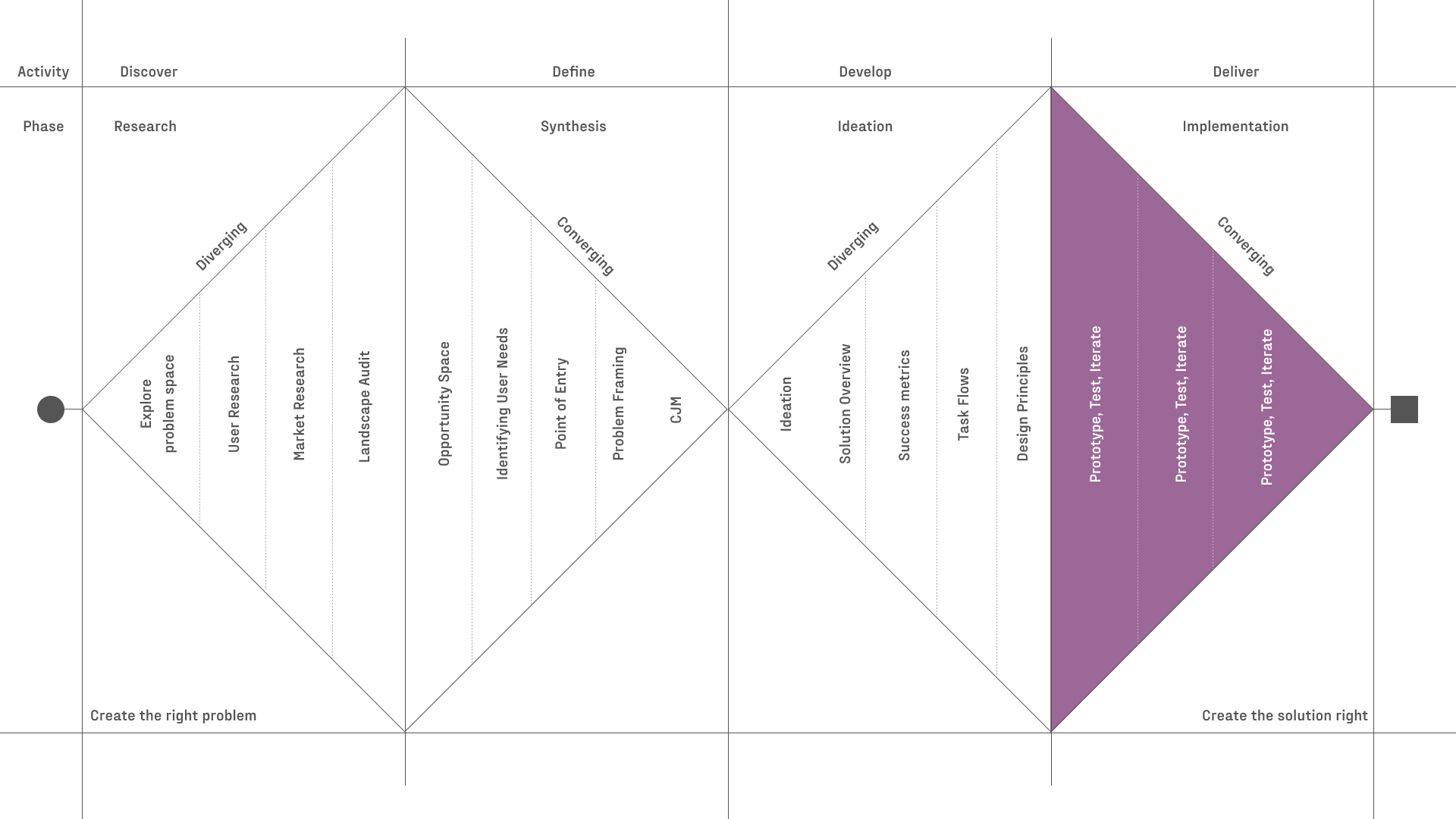
DELIVER
MVP Solutions
The following solutions were designed and implemented with a focus on both developers and the established design principles, while ensuring the appropriate integration of research insights. Working collaboratively with developers allowed for rapid iteration, testing, and refinement.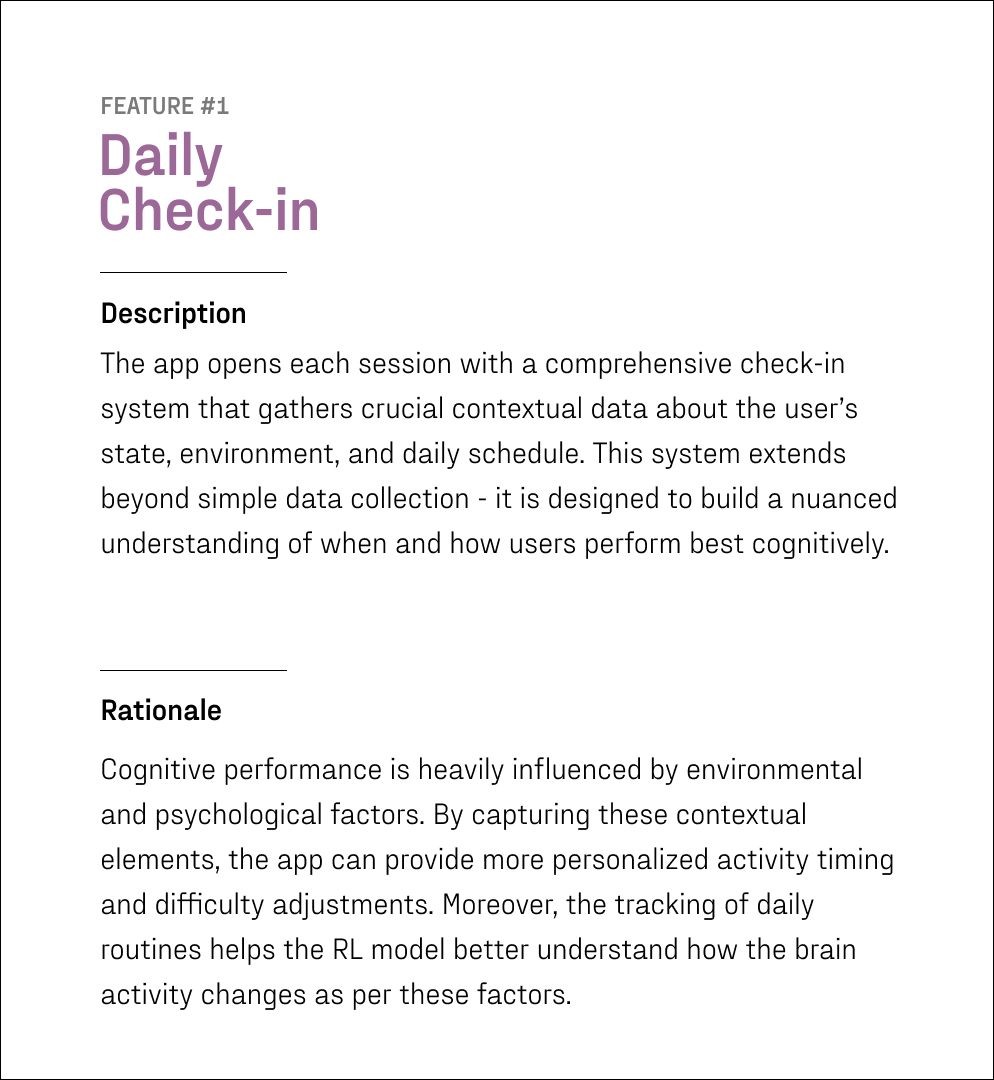
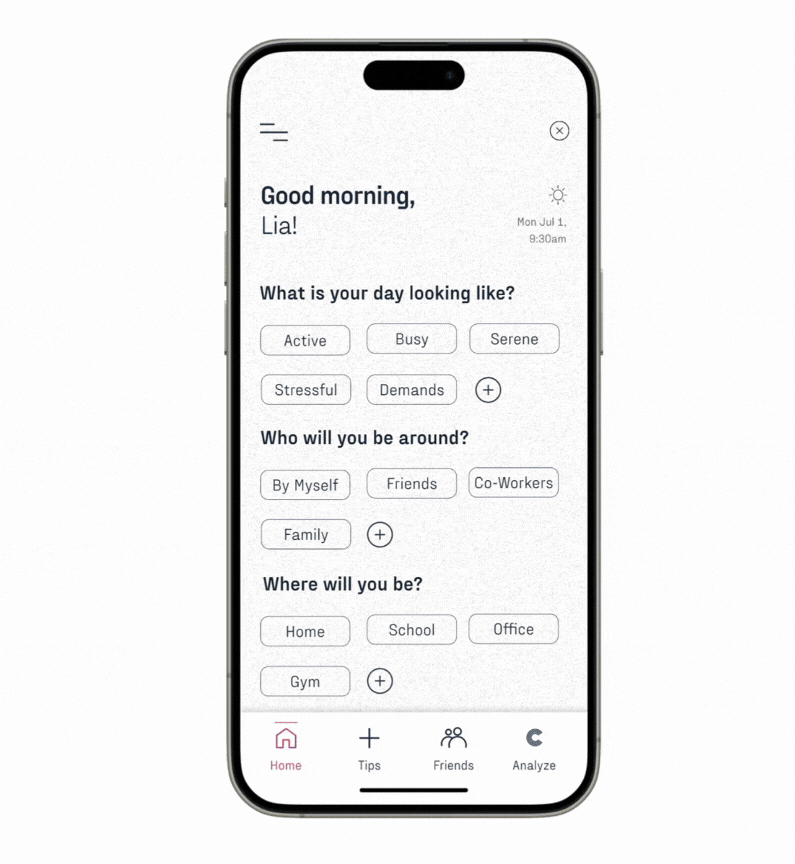
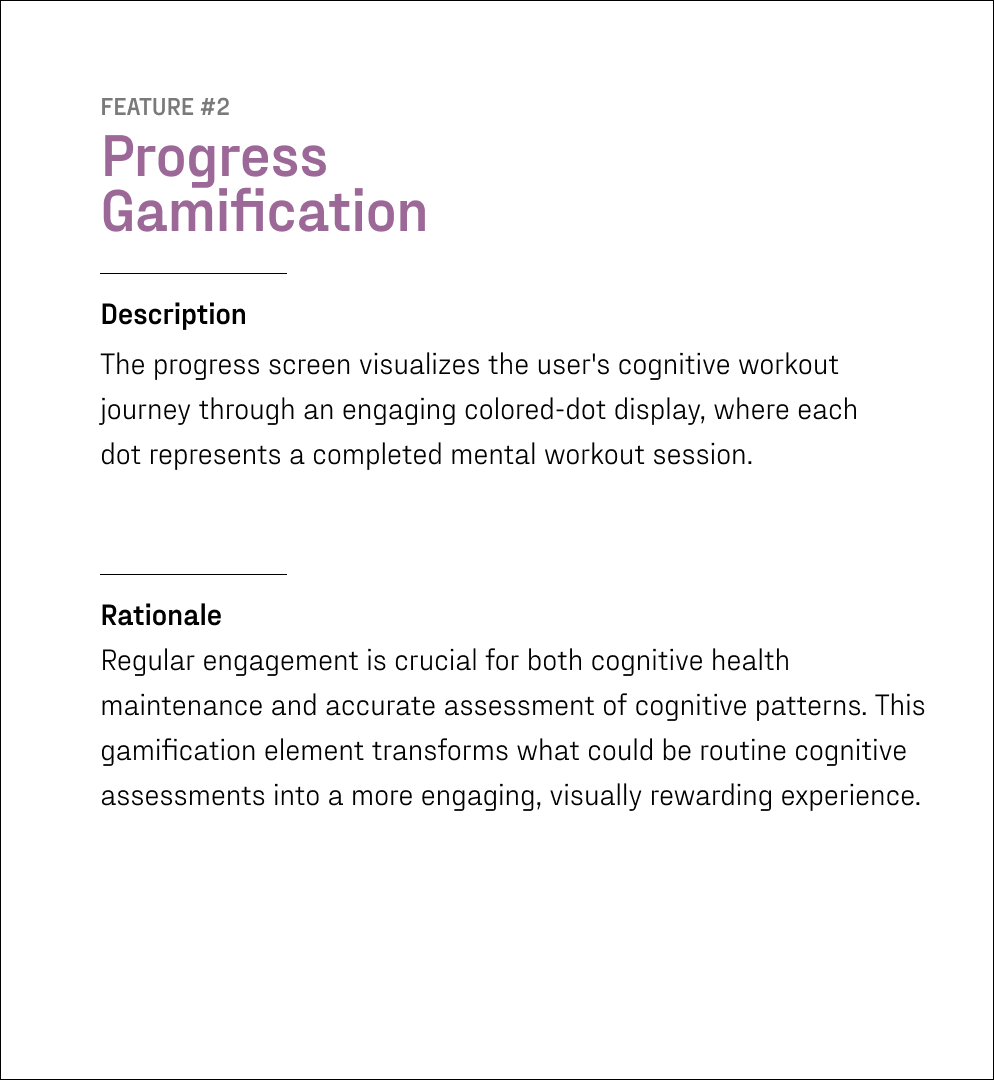
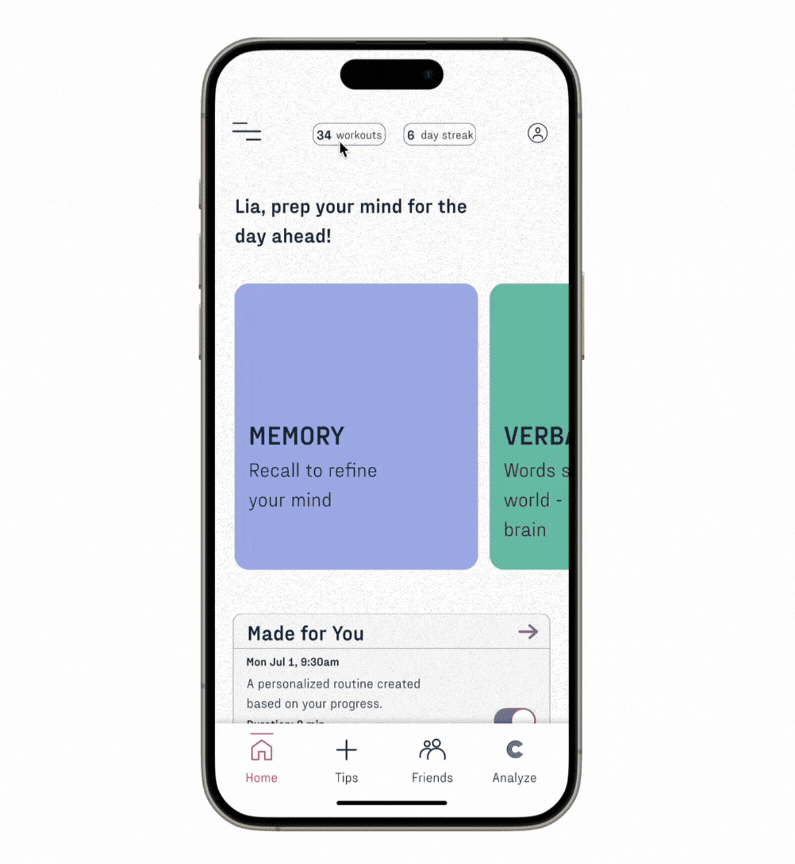
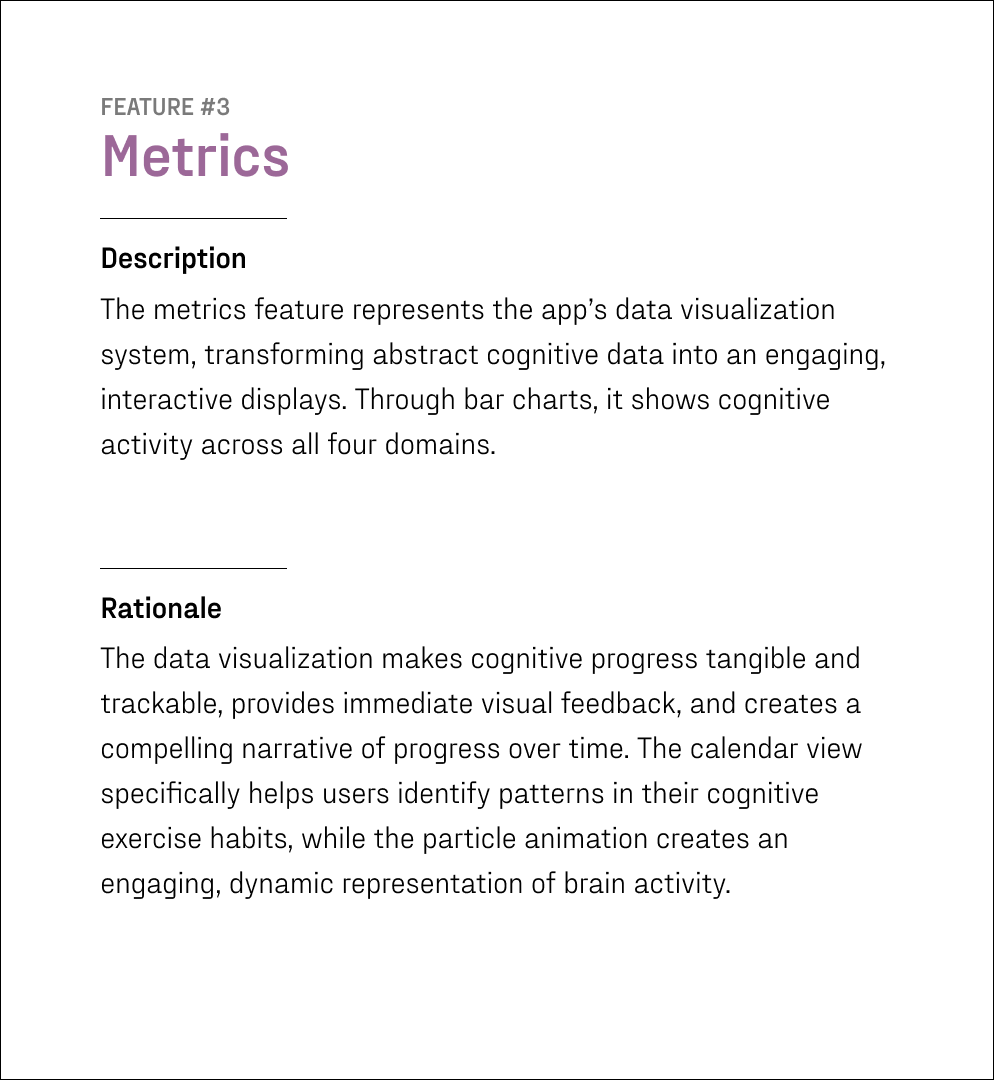
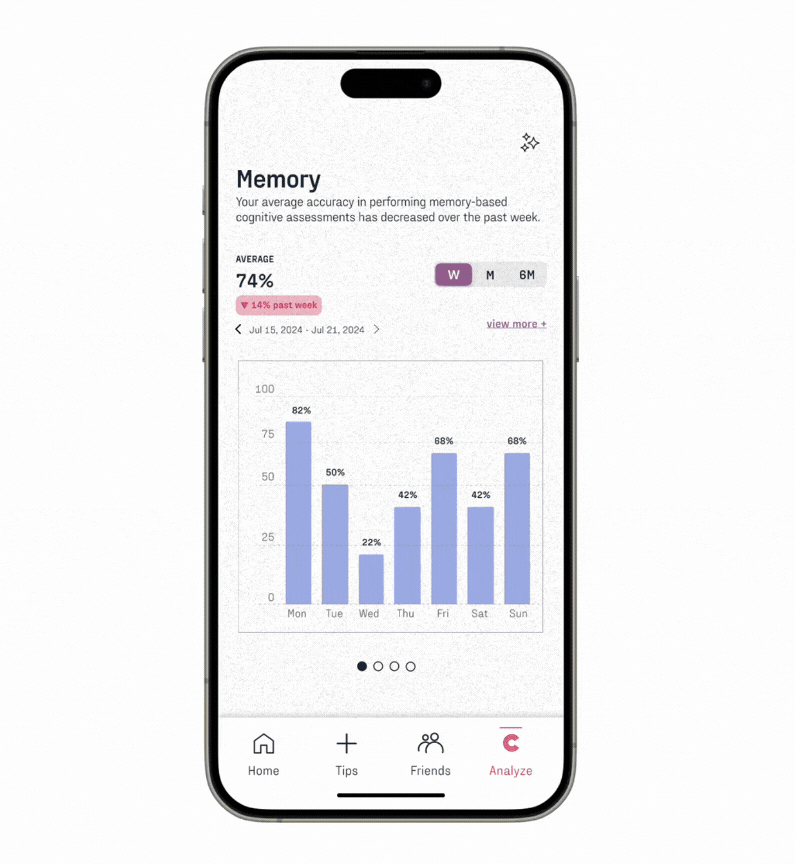
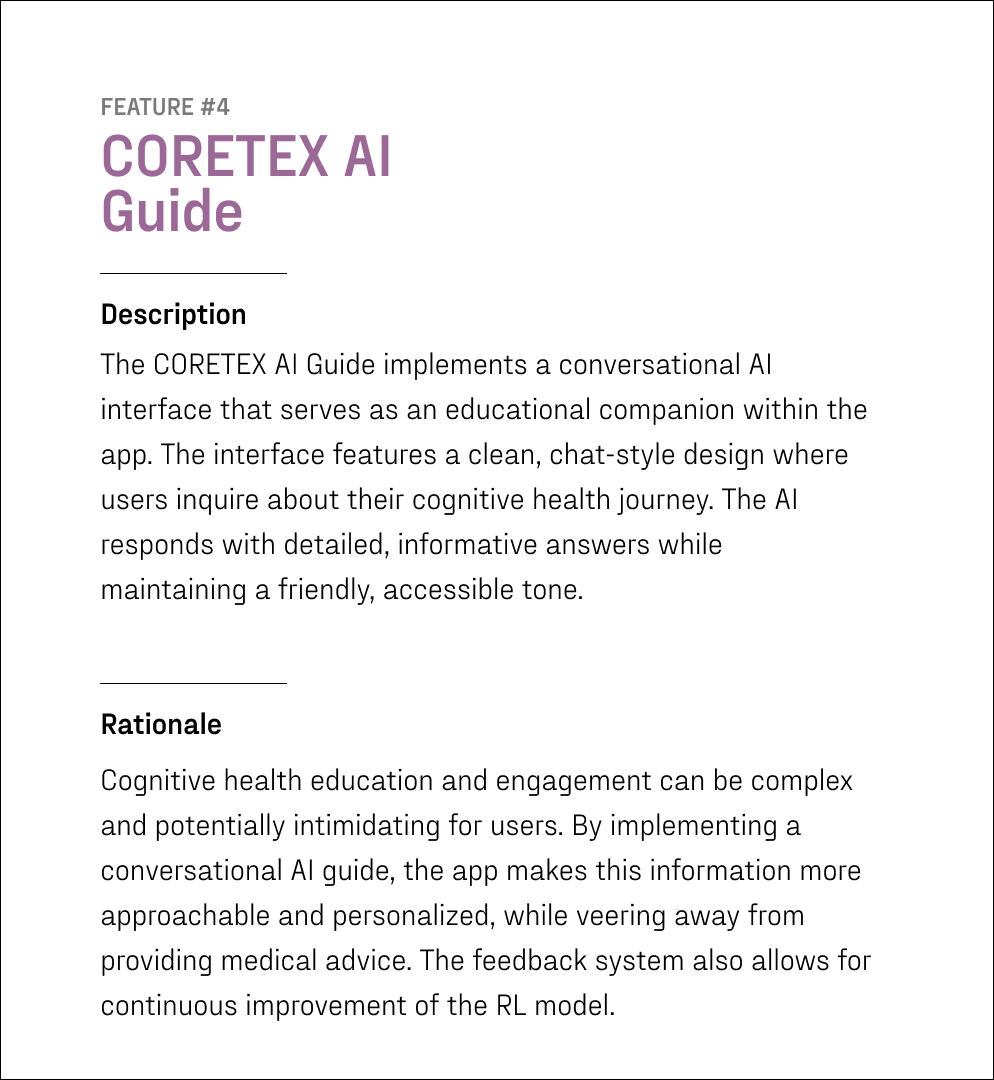
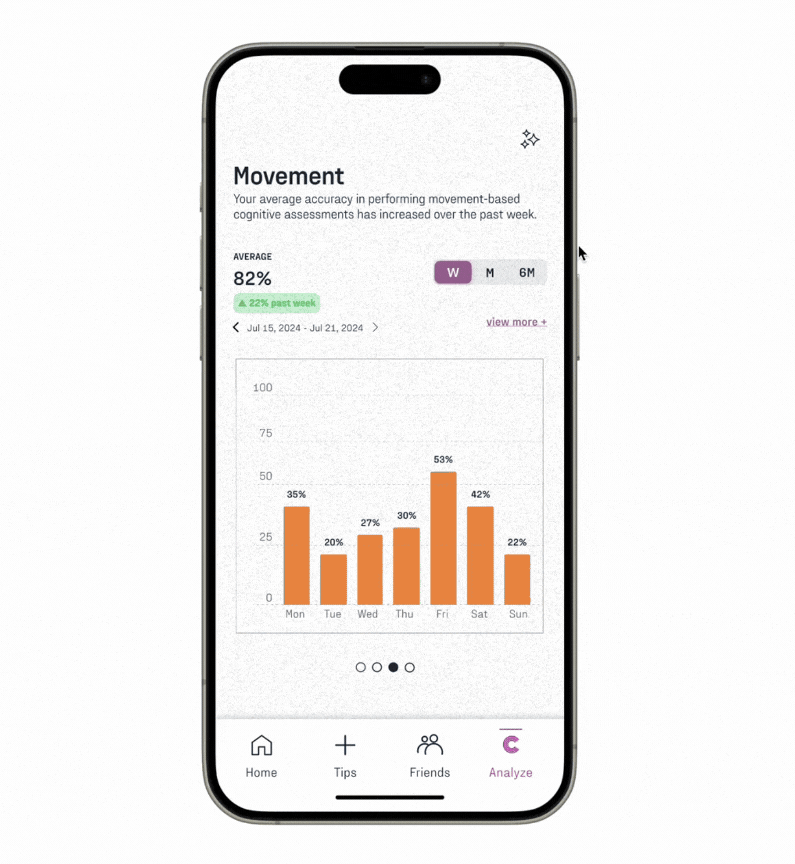
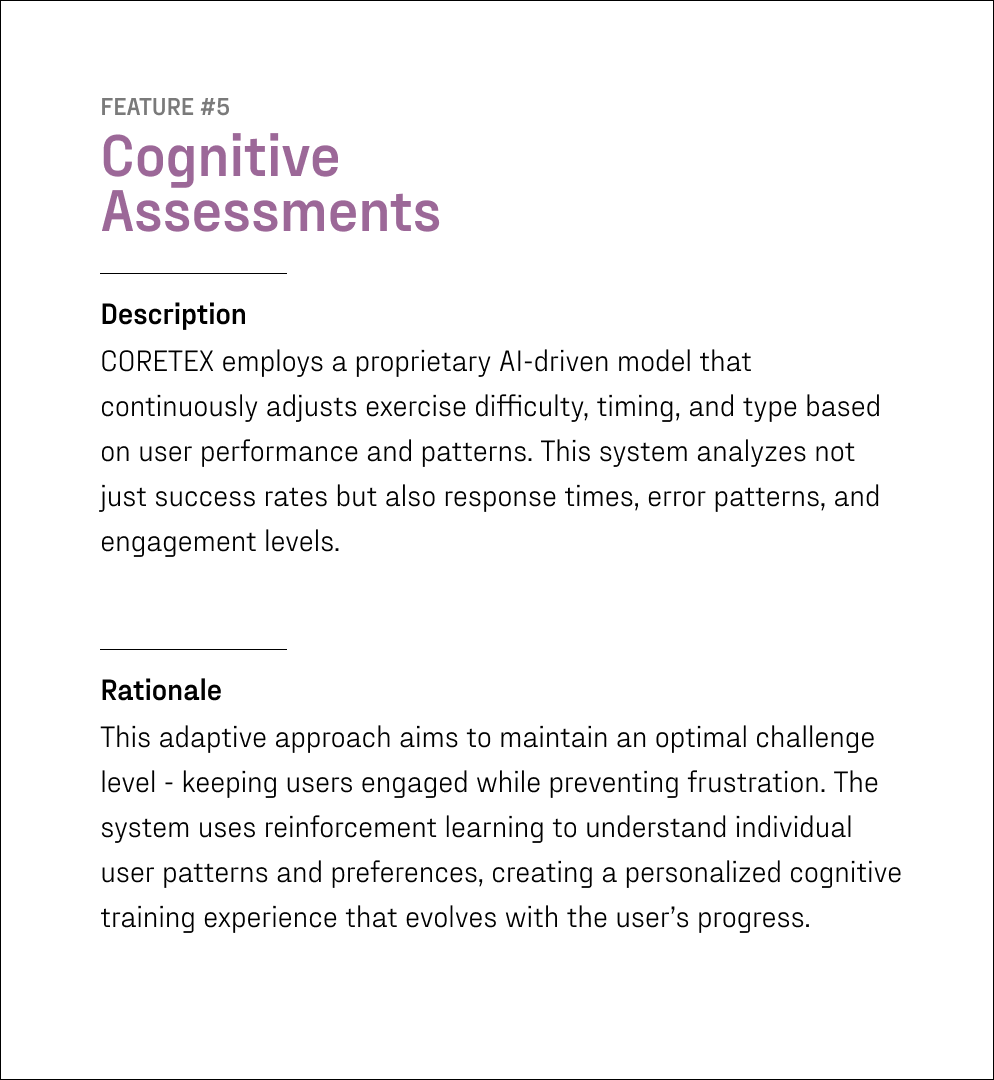
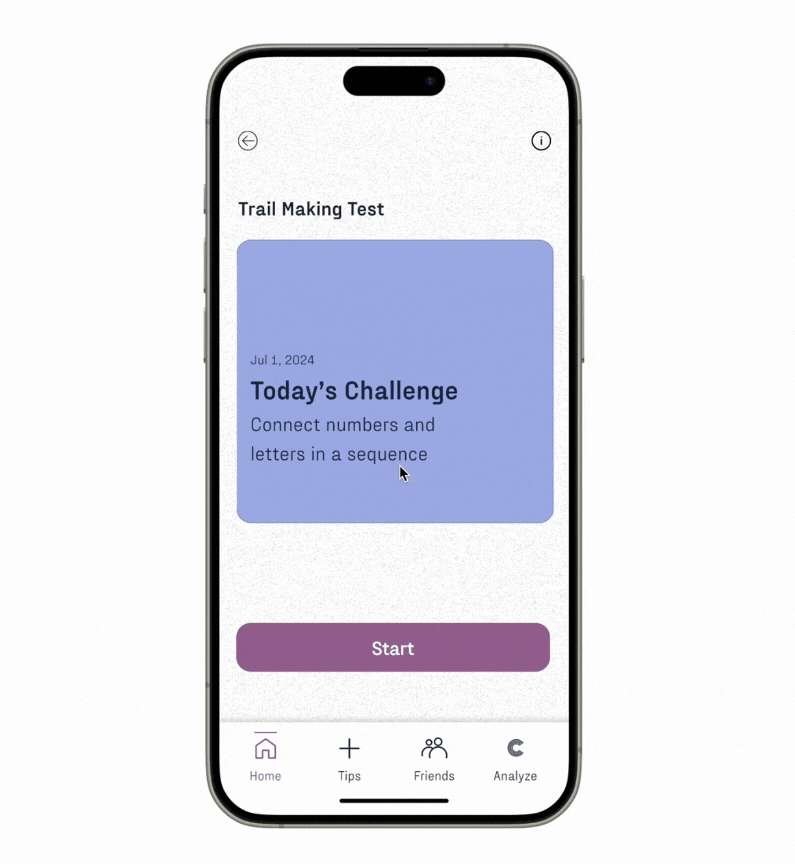
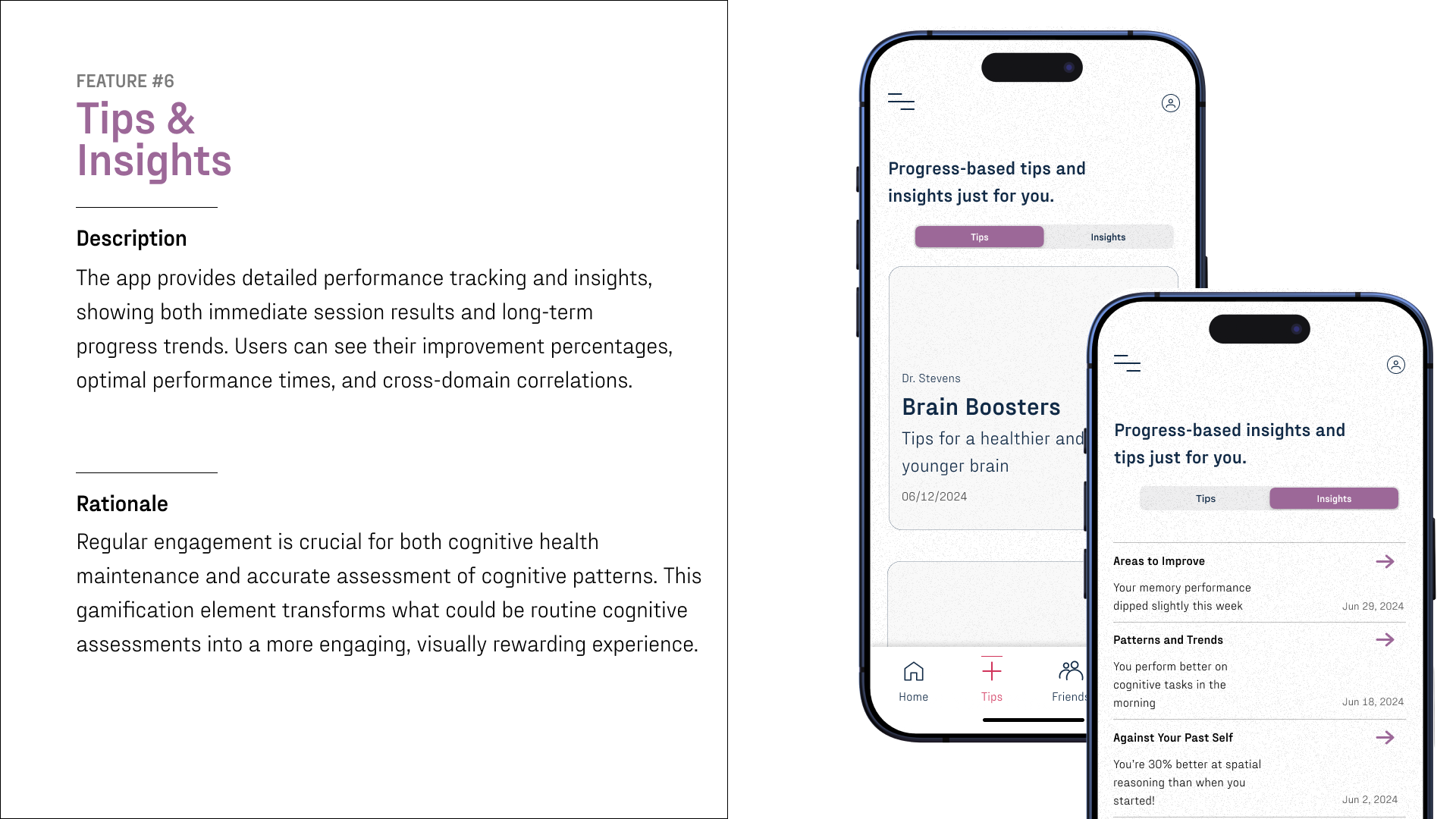
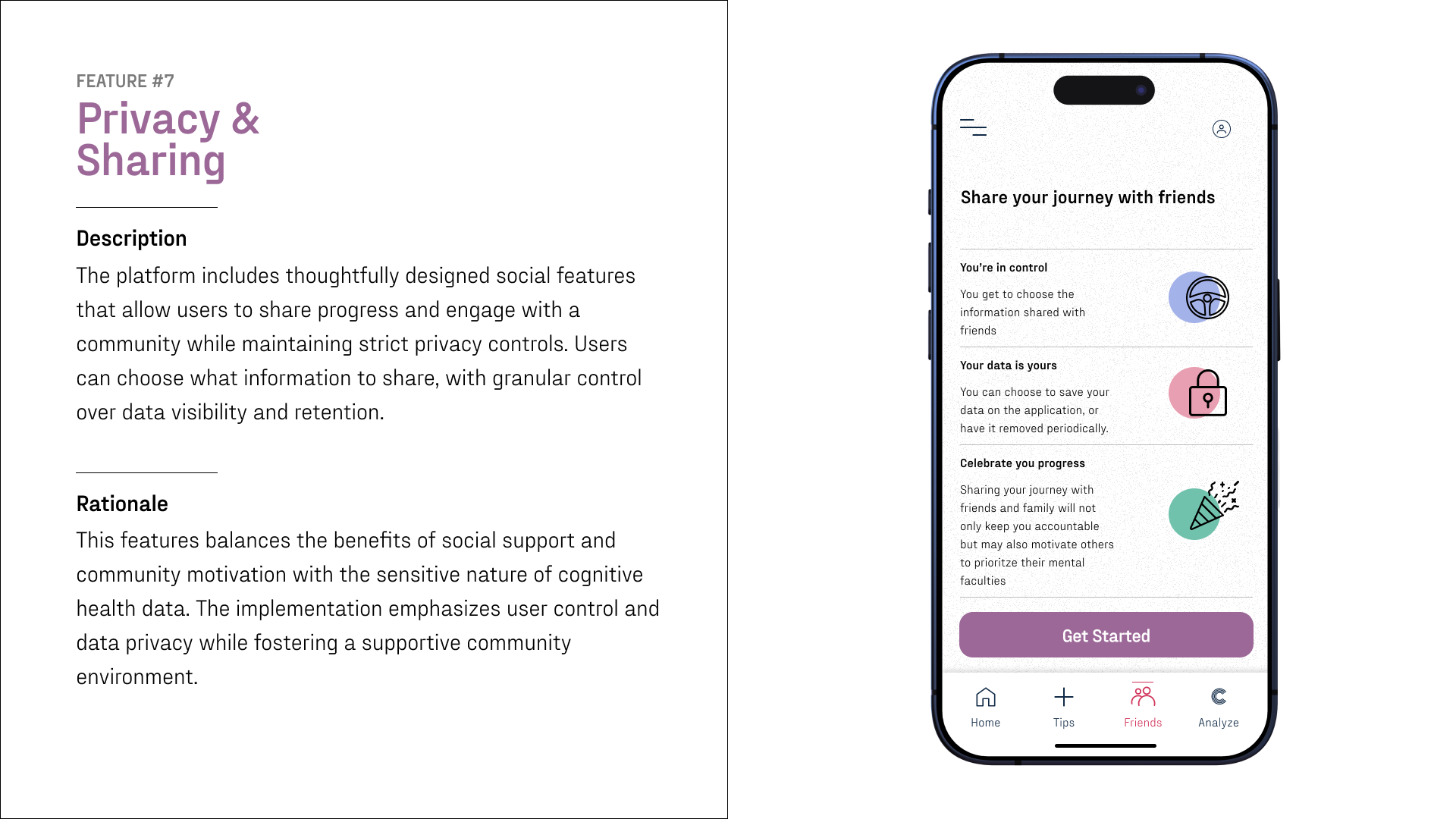
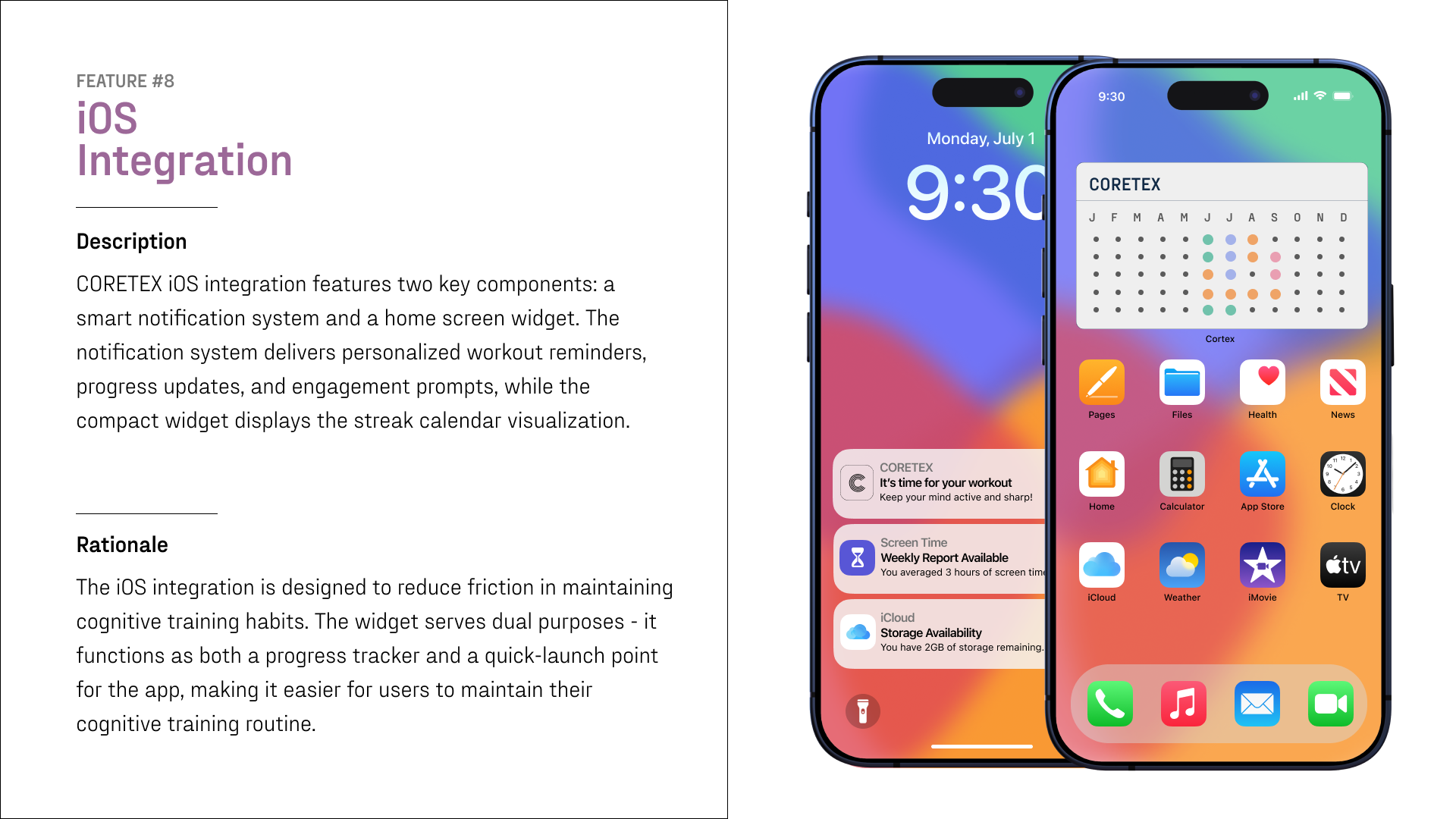
Closing Thoughts
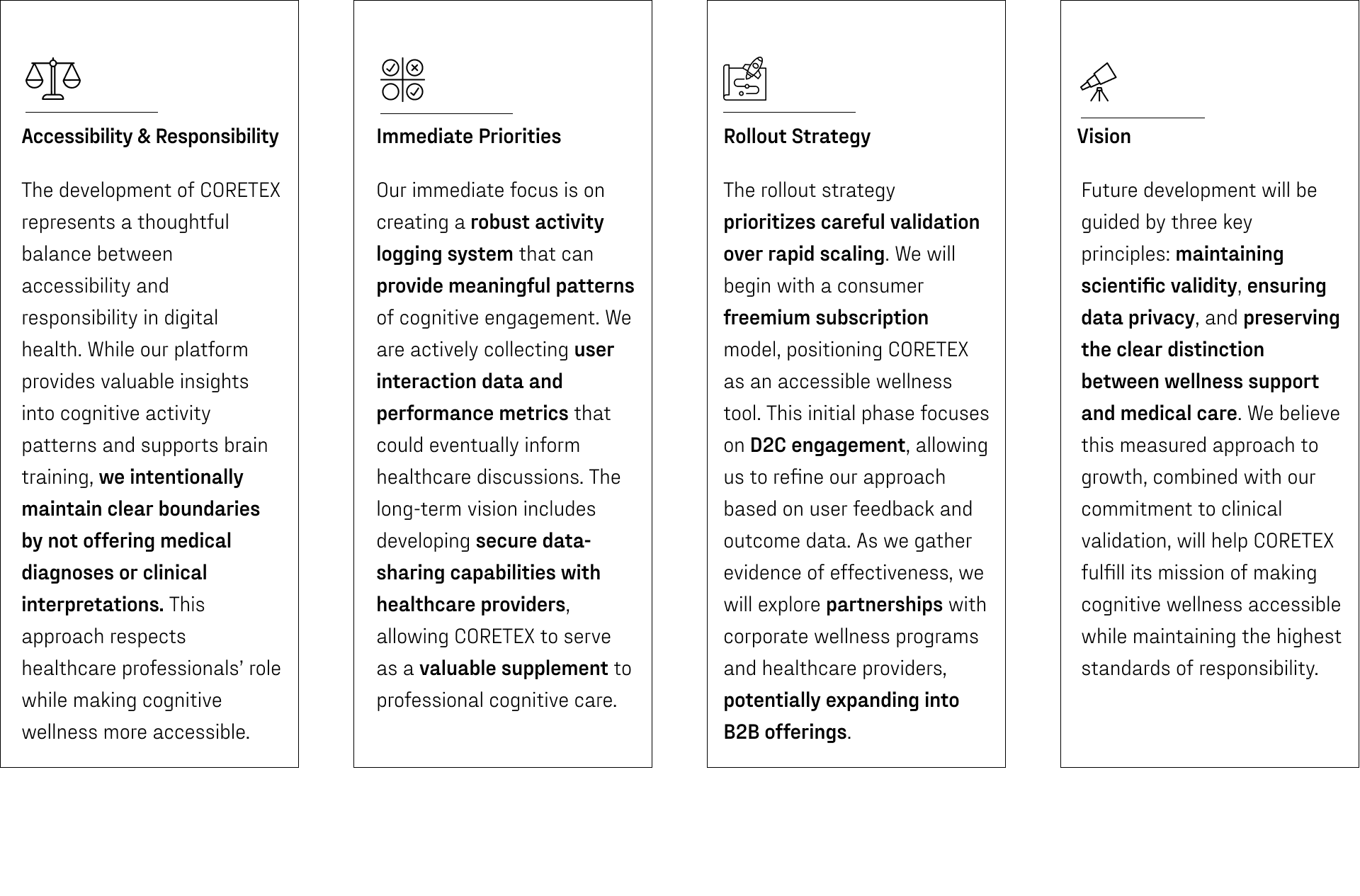
